Automated Data Extraction and its Role in Transforming Business
Although there is substantial digitization for exchanging information within an organization, such information is still shared in its physical form via paper when communicating with customers and other business parties. As a physical text, there is still a large amount of legacy material, and if it were converted to digital, this would have enormous value.
Traditionally, a wide pool of human staff read, infer, and extract information from these documents as part of organizational processes driven by IT automation which feeds into the operational systems for further processing. We have entered an age of automated information or data extraction with the emergence of RPA, Machine Learning, and AI technology that leverages intelligent automation solutions. These innovations have immense potential for transforming the way companies function and do business.
Document Varieties and Their Formats
Most of the physical document information is scanned using scanner machines and stored in pdf or other image formats such as jpg, png, or tiff. If the information is not too old and legacy-driven, a standard text in pdf format can also exist (searchable). This includes free-flow text or paragraphs, mark and value pairs, tables, charts or figures or photographs, bar codes, text printed on stamps, smaller picture areas such as emblem or signature, illustrations, handwritten text, among many other such items. The representative set of document forms dealt with by companies are:
- Contracts: all types of legal agreements are included. Typically, extracting key metadata attributes, unique text clauses, and inference-based results are needed. It may also require inferring on a clause which particular group/s within the organization ought to behave.
- Proof of identity/revenue/address documents: Paperwork is needed to extract critical details about an individual or organization. Based on country, state, area, layouts, and formats can vary.
- Transactional records: These include documents such as invoices, instructions for work, etc. The format and layout can be variable or set.
- Table-based documents: Certain documents are table-based, such as price sheets, product descriptions, etc., and all the information in the table needs to be collected and supplied to other downstream systems.
- Drawing documents: These include diagrammatic representations such as maps of the geographical area, sketches of viewpoints, or other such drawings. The necessity may be to remove only some elements of labels and values from the picture, or it may be as complex as extracting much of the drawing’s details to be replicated in another method.
- Pre-print input types: Many application/survey forms contain pre-printed instructions, enabling users to input information for enterprise intelligent automation as numbers and characters inboxes and combs or allow free handwriting.
- Product images: Details such as brand name, weight or amount, nutritional data, ingredients, etc., must be read from product packaging images several times.
The Role of Foundational Technology
Intelligent Automation Services makes it possible to understand the document’s structure, the document’s material, associated labels, and their values, and to extract critical data from these documents in turn.
These components of technology are accessible as paid or open-source applications and include:
Computer Vision: Each page of a scanned document is an image. Intelligent automation companies use vision software libraries that are first used to understand and classify any image variable of interest, such as paragraphs, tables, logos, handwritten text, boxes, etc., using thresholding techniques, contouring, etc.
Optical Character Recognition: OCR libraries extract all the text characters present in the area until a region of interest is identified. Such libraries offer an AI model trained with character sets of various fonts and sizes of a vast number of forms.
Natural Language Processing: NLP is used to translate the contract’s language when, as part of any agreement, material in the form of clauses is present. Intelligent automation solutions can define individuals and their beliefs. Open-source libraries are common, such as Spacy, NLTK, RASA, etc., that are pre-trained in interpretation and can also be trained to extract values from trained entities.
Intelligent Character Recognition: ICR technology is used for the identification of the handwritten character. It comprises AI models trained with large quantities of training data for handwritten text pictures and annotated real text values. Although businesses sell ICR products, an existing pre-trained open-source model may be used for particular problems, and specific training may be added in addition to it. Data extraction uses a combination of the above foundational technology components.
Challenges in Data Extraction
Documents are available in image form in most cases. Many noise and quality elements usually pose a challenge to the extraction of information and data transfer to a digital format. These include watermarks, pen scribbles, wrinkled, ripped, decolored, smudged, stamps printed on the text, black and white grains randomly occurring, dark backgrounds, fading ink, low-contrast or colored ink printed, scribbling on printed text, weak scan dpi.
With fused and spilled cells, rotated tables, undefined borders, and several other derivatives, tables can or may not have grid lines and can get complex. Also, cursive writing and no simple distinction of characters in handwriting make it hard to extract data from hand-written text. Another difficulty when processing legal documents is deriving inference from several similar sentences in a clause or section. It has its challenges to consider the layout and placements of crucial information in documents that do not have a set format, such as an invoice, and extract data from those.
This is why we need another layer of extraction solution beyond simple technologies that can solve specific problems in data extraction. To check and correct extracted information, a business-friendly workbench adds a great deal of value. In the next blog of this extraction series, we will look at elements of an extraction approach to solve the above problems. We look at potential developments in data extraction and digital data transfer.
Share This Post
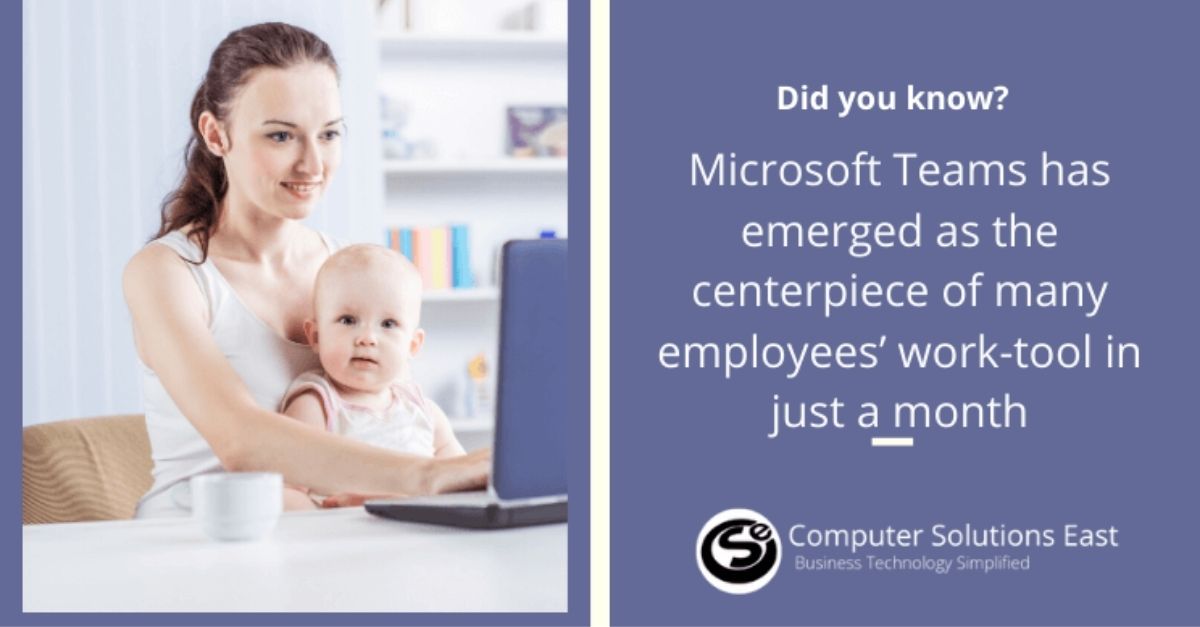